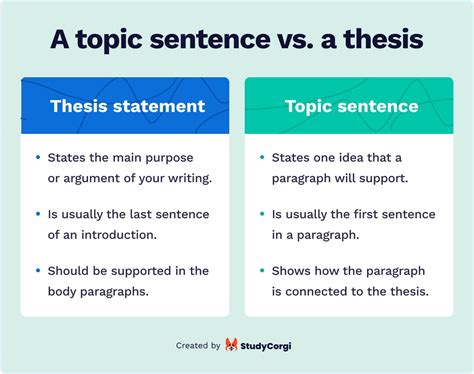
Adding a best fit line to your Excel scatterplot can be a valuable tool for understanding the relationship between your data points. By calculating the slope and intercept of the line, you can determine the overall trend of your data and make predictions about future values. This article will provide a step-by-step guide to adding a best fit line in Excel, ensuring you can easily extract insights from your data.
To begin, you will need to select the scatterplot on your Excel worksheet. Once selected, click the “Insert” tab in the ribbon menu and choose “Chart Elements” > “Trendline.” From the drop-down menu, select “Linear” to add a straight line to your data. If desired, you can customize the line style, color, and weight to match the aesthetics of your chart. Excel will automatically calculate the slope and intercept of the line, which will be displayed on the chart.
The slope of the best fit line represents the change in the y-value for every one-unit change in the x-value. For example, if the slope is 2, then the y-value will increase by 2 for every one-unit increase in the x-value. The intercept, on the other hand, represents the value of y when x is equal to zero. By understanding the slope and intercept of the best fit line, you can draw conclusions about the relationship between your data points. Additionally, you can use the line to make predictions about future values by plugging in different x-values into the equation of the line (y = mx + b, where m is the slope and b is the intercept).
Understanding the Best Fit Line
A best fit line is a straight line that most accurately represents the trend of a set of data points. It is a statistical tool used to describe the relationship between two or more variables. The best fit line is calculated using a statistical technique called linear regression, which determines the line that minimizes the sum of the squared distances between the data points and the line.
The best fit line has the following properties:
- The slope of the line indicates the rate of change of the y-variable with respect to the x-variable.
- The y-intercept of the line indicates the value of the y-variable when the x-variable is zero.
- The line passes through the centroid of the data points, which is the average of all the data points.
The best fit line is used to predict the value of the y-variable for a given value of the x-variable. It is also used to test the significance of the relationship between the two variables and to determine the correlation between them.
Term |
Definition |
Slope |
The rate of change of the y-variable with respect to the x-variable. |
Y-intercept |
The value of the y-variable when the x-variable is zero. |
Centroid |
The average of all the data points. |
Calculating the Regression Equation
The regression equation is a mathematical equation that describes the relationship between a dependent variable and one or more independent variables. In the case of a best-fit line, the dependent variable is the y-value and the independent variable is the x-value. The equation takes the form:
“`
y = mx + b
“`
where:
- y is the dependent variable
- x is the independent variable
- m is the slope of the line
- b is the y-intercept
To calculate the regression equation, we need to find the values of m and b. This can be done using the following formulas:
“`
m = (∑(x – x̄)(y – ȳ)) / (∑(x – x̄)²)
“`
“`
b = ȳ – m * x̄
“`
where:
- x̄ is the mean of the x-values
- ȳ is the mean of the y-values
Once we have calculated the values of m and b, we can plug them into the regression equation to get the equation for the best-fit line.
For example, let’s say we have the following data:
We can use the formulas above to calculate the regression equation for this data. First, we calculate the means of the x-values and y-values:
“`
x̄ = (1 + 2 + 3) / 3 = 2
ȳ = (2 + 4 + 6) / 3 = 4
“`
Next, we calculate the slope of the line:
“`
m = ((1 – 2)(2 – 4) + (2 – 2)(4 – 4) + (3 – 2)(6 – 4)) / ((1 – 2)² + (2 – 2)² + (3 – 2)²) = 1
“`
Finally, we calculate the y-intercept:
“`
b = 4 – 1 * 2 = 2
“`
Therefore, the regression equation for the best-fit line is:
“`
y = x + 2
“`
Using the LINEST() Function
The LINEST() function in Excel is a powerful tool for performing linear regression analysis. It allows you to determine the best-fit line for a set of data, which can be used to make predictions or draw conclusions about the relationship between the variables.
The syntax of the LINEST() function is as follows:
“`
=LINEST(y_range, x_range, [const], [stats])
“`
where:
- y_range is the range of cells containing the dependent variable (the variable you are trying to predict).
- x_range is the range of cells containing the independent variable (the variable that you are using to make the prediction).
- const (optional) is a logical value (TRUE or FALSE) that indicates whether or not to include a constant term in the regression equation. If TRUE, a constant term will be included; if FALSE, no constant term will be included.
- stats (optional) is a logical value (TRUE or FALSE) that indicates whether or not to return additional statistical information about the regression. If TRUE, the LINEST() function will return an array of values containing the following information:
Element |
Description |
1 |
Slope of the regression line |
2 |
Intercept of the regression line |
3 |
Standard error of the slope |
4 |
Standard error of the intercept |
5 |
R-squared statistic |
6 |
F-statistic |
7 |
Degrees of freedom for the numerator |
8 |
Degrees of freedom for the denominator |
9 |
Mean of the y-values |
10 |
Mean of the x-values |
To use the LINEST() function, simply enter the following formula into a cell:
“`
=LINEST(y_range, x_range, [const], [stats])
“`
where you replace y_range and x_range with the ranges of cells containing your data. If you want to include a constant term in the regression equation, enter TRUE for the const argument. If you want to return additional statistical information, enter TRUE for the stats argument.
Interpreting the Slope and Y-Intercept
The slope and y-intercept provide valuable insights into the relationship between the variables represented in the scatter plot. Here’s a detailed explanation of each:
Slope
The slope of a linear regression line measures the change in the dependent variable (y-axis) for each unit change in the independent variable (x-axis). A positive slope indicates a direct relationship, while a negative slope indicates an inverse relationship. The magnitude of the slope represents the steepness of the line.
Example:
In a scatter plot showing the relationship between height and weight, a slope of 0.5 implies that for each additional inch of height, the weight increases by 0.5 pounds.
Y-Intercept
The y-intercept is the value of the dependent variable when the independent variable is zero. It represents the starting point of the regression line on the y-axis. A positive y-intercept indicates that the line crosses the y-axis above the origin, while a negative y-intercept indicates that it crosses below.
Example:
If the y-intercept of a line in a scatter plot showing the relationship between height and weight is 50 pounds, it means that even if someone has zero height, their predicted weight is 50 pounds.
Slope |
Y-Intercept |
Meaning |
Positive |
Positive |
Direct relationship, starting above the origin |
Negative |
Positive |
Inverse relationship, starting above the origin |
Positive |
Negative |
Direct relationship, starting below the origin |
Negative |
Negative |
Inverse relationship, starting below the origin |
Determining Goodness of Fit Using R-Squared
The R-squared value is a statistical measure that indicates the goodness of fit of a best-fit line to a set of data points. It measures the proportion of variance in the dependent variable that is explained by the independent variable.
Calculating R-Squared
R-squared is calculated using the following formula:
R-squared = 1 – (SSresidual / SStotal)
where:
- SSresidual is the sum of squared residuals, which measures the vertical distance between each data point and the best-fit line.
- SStotal is the sum of squared deviations from the mean, which measures the total variance in the dependent variable.
Interpreting R-Squared
The R-squared value can range from 0 to 1.
A value of 0 indicates that the best-fit line does not explain any variance in the dependent variable, while a value of 1 indicates that the best-fit line perfectly fits the data points.
Uses of R-Squared
R-squared is a useful tool for:
- Evaluating the accuracy of a linear regression model.
- Comparing different linear regression models to determine the one that best fits the data.
- Making predictions about future values of the dependent variable.
Limitations of R-Squared
R-squared should be interpreted cautiously, as it can be influenced by the number of data points and the presence of outliers.
It is important to consider other measures of goodness of fit, such as the adjusted R-squared and the root mean squared error, when evaluating a linear regression model.
Example
Consider the following data:
The best-fit line for this data is y = 2 + x. The R-squared value for this line is 0.98, which indicates that the line explains 98% of the variance in the y-values.
Applying the Best Fit Line to Data Analysis
The best fit line, also known as the regression line, is a graphical representation of the linear relationship between two variables. It helps in understanding the trend in the data and making predictions. There are several types of best fit lines, but the most common is the linear best fit line.
Benefits of Using the Best Fit Line
- Visualize Data: The best fit line provides a visual representation of the relationship between variables, making it easier to identify trends and patterns.
- Predict Values: Using the equation of the line, we can predict values of the dependent variable for given values of the independent variable.
- Identify Outliers: Points that deviate significantly from the best fit line may indicate outliers or measurement errors.
How to Add a Best Fit Line in Excel
Follow these steps to add a best fit line in Excel:
1. Select the data range that contains the independent and dependent variables.
2. Click on the “Insert” tab on the ribbon.
3. In the “Charts” group, click on the “Line” chart icon.
4. Choose a line chart subtype as per your preference.
5. Right-click on a data point in the chart.
6. Select “Add Trendline” from the context menu.
Trendline Options
The “Format Trendline” dialog box provides several options to customize the best fit line:
Option |
Description |
Type |
Select the type of best fit line (e.g., Linear, Exponential, Logarithmic). |
Display Equation on chart |
Check this option to show the equation of the line on the chart. |
Display R-squared value on chart |
Check this option to display the coefficient of determination (R²) on the chart, which measures how well the line fits the data. |
The trendline can be used to interpolate values within the range of the data, or extrapolate values beyond the range of the data. However, it is important to use caution when extrapolating, as the predictions may not be accurate outside the observed range.
Forecasting Future Values with the Best Fit Line
7. Determining the Slope and Y-Intercept
The slope of the best fit line represents the rate of change in the dependent variable (y) for each unit change in the independent variable (x). To calculate the slope, use the formula:
“`
slope = (Σ(x – x̄)(y – ȳ)) / (Σ(x – x̄)²)
“`
where:
– Σ is the sum of the values
– x̄ is the mean of the x values
– ȳ is the mean of the y values
The y-intercept represents the value of y when x is equal to zero. To calculate the y-intercept, use the formula:
“`
y-intercept = ȳ – slope * x̄
“`
Once you have determined the slope and y-intercept, you can write the equation of the best fit line:
“`
y = slope * x + y-intercept
“`
Using this equation, you can predict future values for y based on any given x value. For example, if you have a best fit line for sales data, you can use it to forecast future sales based on different levels of investment in advertising.
|
Formula |
Slope |
(Σ(x – x̄)(y – ȳ)) / (Σ(x – x̄)²) |
Y-Intercept |
ȳ – slope * x̄ |
Visualizing the Best Fit Line in Excel
Add a Best Fit Line to a Scatter Plot
To add a best fit line to a scatter plot, first select the chart. Then, click the “Chart Elements” button in the “Chart Tools” tab, and select “Trendline.” In the “Trendline Options” dialog box, select the type of best fit line you want to add, such as linear, logarithmic, or exponential.
Format the Best Fit Line
Once you have added a best fit line, you can format it to change its color, thickness, or style. To do this, right-click the best fit line and select “Format Trendline.” In the “Format Trendline” dialog box, you can make changes to the line’s appearance.
Show or Hide the Best Fit Line Equation
You can also show or hide the equation of the best fit line. To do this, right-click the best fit line and select “Add Trendline Equation.” If the equation is already visible, you can hide it by selecting “Remove Trendline Equation.”
Use the Best Fit Line to Make Predictions
Once you have added a best fit line, you can use it to make predictions. To do this, select a point on the scatter plot and drag it to a new location. The best fit line will automatically update, and the equation of the best fit line will change to reflect the new data.
Customizing the Best Fit Line
You can also customize the best fit line by changing the intercept or slope of the line. To do this, right-click the best fit line and select “Format Trendline.” In the “Format Trendline” dialog box, you can change the intercept or slope of the line.
Removing the Best Fit Line
To remove the best fit line, right-click the best fit line and select “Delete Trendline.”
Error Bars on Best Fit Lines
You can add error bars to a best fit line to show the uncertainty in the data. To do this, right-click the best fit line and select “Add Error Bars.” In the “Format Error Bars” dialog box, you can choose the type of error bars you want to add.
Table of Best Fit Line Options
Option |
Description |
Linear |
A straight line that best fits the data |
Logarithmic |
A curved line that best fits the data |
Exponential |
A curved line that best fits the data |
Polynomial |
A curved line that best fits the data |
Moving Average |
A line that shows the average of the data over a specified number of periods |
Analyzing Trends and Patterns Using the Best Fit Line
The best fit line is a valuable tool for analyzing trends and patterns in data. By fitting a straight line to a set of data points, we can gain insights into the overall trend of the data and identify any outliers or patterns. Here are the steps involved in adding a best fit line to your data in Excel:
- Select the data points you want to analyze.
- Click on the “Insert” tab in the Excel menu.
- In the “Charts” section, select the “Scatter” chart type.
- Once the chart is inserted, right-click on one of the data points and select “Add Trendline”.
- In the “Trendline Options” dialog box, select the “Linear” trendline type.
- Check the “Display Equation on chart” box to display the equation of the best fit line on the chart.
- Click “OK” to add the best fit line to your chart.
Once you have added a best fit line to your chart, you can use it to:
- Estimate the value of y for a given value of x.
- Identify the slope and y-intercept of the line.
- Determine the correlation coefficient between x and y.
The Equation of the Best Fit Line
The equation of the best fit line is a linear equation in the form y = mx + b, where m is the slope of the line and b is the y-intercept. The slope represents the change in y for each unit change in x, and the y-intercept represents the value of y when x = 0. You can use the equation of the best fit line to make predictions about the value of y for future values of x.
The Correlation Coefficient
The correlation coefficient is a measure of the strength of the linear relationship between x and y. It can range from -1 to 1, where -1 indicates a perfect negative correlation, 0 indicates no correlation, and 1 indicates a perfect positive correlation. A correlation coefficient close to 0 indicates that there is no linear relationship between x and y, while a correlation coefficient close to 1 indicates a strong linear relationship. You can use the correlation coefficient to determine how well the best fit line fits the data.
Correlation Coefficient |
Interpretation |
-1 to -0.7 |
Strong negative correlation |
-0.6 to -0.3 |
Moderate negative correlation |
-0.2 to 0.2 |
Weak correlation |
0.3 to 0.6 |
Moderate positive correlation |
0.7 to 1 |
Strong positive correlation |
Limitations of the Best Fit Line
While the best fit line can provide valuable insights, it has certain limitations:
- Data Range and Extrapolation: The best fit line assumes a linear relationship within the given data range. Extrapolating beyond the data range can lead to inaccurate predictions.
- Non-Linearity: The best fit line is linear, but the underlying relationship between the variables may not always be linear. In such cases, a different type of curve fitting may be required.
- Outliers: Extreme data points (outliers) can significantly distort the best fit line. It’s important to identify and handle outliers appropriately.
- Correlation does not imply Causation: A strong correlation between variables does not necessarily indicate a causal relationship. Other factors may be influencing the relationship.
Considerations for the Best Fit Line
When using the best fit line, it’s crucial to consider the following:
10. Goodness-of-Fit Statistics
Evaluate the goodness-of-fit through statistics like the coefficient of determination (R-squared), root mean squared error (RMSE), and adjusted R-squared. These metrics indicate how well the line fits the data.
Goodness-of-Fit Statistic |
Description |
R-squared |
The proportion of the variability in the dependent variable that is explained by the independent variable. |
RMSE |
The average distance between the data points and the best fit line. |
Adjusted R-squared |
An R-squared value that has been adjusted to account for the number of independent variables in the model. |
Add Best Fit Line Excel
Introduction
Adding a best fit line to your Excel data can help you visualize the relationship between two variables and make predictions about future values. Here are step-by-step instructions on how to do it:
Instructions
1. Select the data range that you want to add a best fit line to.
2. Click on the “Insert” tab.
3. In the “Charts” group, click on the “Scatter” button.
4. Select the “Scatter with Lines” chart type.
5. Click on the “OK” button.
Your chart will now include a best fit line. The line will be displayed in a different color than your data points.
Additional Options
You can customize the appearance of your best fit line by right-clicking on it and selecting the “Format Data Series” option. In the “Format Data Series” dialog box, you can change the line color, weight, and style.
You can also add a trendline equation to your chart by right-clicking on the best fit line and selecting the “Add Trendline” option. In the “Add Trendline” dialog box, you can select the type of equation that you want to add to your chart.
People Also Ask About Add Best Fit Line Excel
How do I add a best fit line without creating a chart?
You can use the SLOPE() and INTERCEPT() functions to add a best fit line to your data without creating a chart. The SLOPE() function calculates the slope of the line, and the INTERCEPT() function calculates the y-intercept of the line.
How do I change the color of the best fit line?
You can change the color of the best fit line by right-clicking on it and selecting the “Format Data Series” option. In the “Format Data Series” dialog box, you can change the line color, weight, and style.
How do I add a trendline equation to my chart?
You can add a trendline equation to your chart by right-clicking on the best fit line and selecting the “Add Trendline” option. In the “Add Trendline” dialog box, you can select the type of equation that you want to add to your chart.