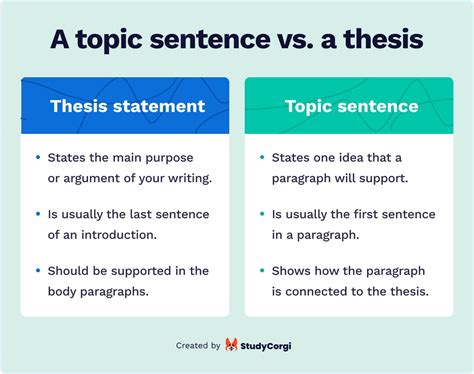
Delving into the realm of data analysis, Excel emerges as an indispensable tool. Its versatile capabilities extend to organizing, summarizing, and presenting data effectively, making it the preferred choice for professionals across various industries. One essential technique in this domain is the frequency table, which provides a concise overview of the distribution of data points. By utilizing Excel’s robust features, creating a frequency table becomes a streamlined and efficient process, enabling you to extract meaningful insights from your data effortlessly.
To embark on this data exploration journey, begin by importing your data into an Excel spreadsheet. Ensure that the data is structured in a single column, with each cell representing a unique data point. Next, select the ‘Data’ tab from the Excel ribbon and navigate to the ‘Data Tools’ group. Click on ‘Frequency’ to invoke the ‘Frequency’ dialog box, which serves as the gateway to creating your frequency table. Within this dialog box, designate the input range by highlighting the column containing your data points and click ‘OK’ to generate the frequency table.
Excel swiftly generates the frequency table, displaying the unique values encountered in your data along with their corresponding frequencies. This table provides a valuable snapshot of the distribution of your data, allowing you to identify the most frequently occurring values and assess the spread of your data. Additionally, you can leverage Excel’s charting capabilities to visualize the frequency distribution graphically, presenting your findings in an engaging and visually impactful manner.
What is a Frequency Table?
A frequency table is a way of organising raw data to show you the frequency of occurrence of different values. It shows how many times a specific value appears in a data set. Frequency tables are useful for data analysis because they can help you to identify patterns, trends, and outliers. Another name for a frequency table is a frequency distribution. Frequency tables are typically used in descriptive statistics. Creating a frequency table can be an easy way to summarise a large amount of data quickly. It will show you the values in your data set, as well as how often each value occurs. For example, if you are analysing the age of customers in a shop, you could create a frequency table to show the number of customers in each age group.
Frequency tables can be created for both qualitative and quantitative data. Quantitative data is data that can be measured, such as age or height. Qualitative data is data that cannot be measured, such as gender or occupation. In a frequency table for qualitative data, the values are the different categories of data. In a frequency table for quantitative data, the values are the different ranges of data.
Here is an example of a frequency table for qualitative data:
Hair Color |
Frequency |
Blonde |
10 |
Brunette |
15 |
Red |
5 |
This table shows that there are 10 blonde people, 15 brunette people, and 5 red-haired people in the data set.
Here is an example of a frequency table for quantitative data:
Height Range |
Frequency |
0-10 |
5 |
11-20 |
10 |
21-30 |
15 |
This table shows that there are 5 people in the data set who are between 0 and 10 years old, 10 people who are between 11 and 20 years old, and 15 people who are between 21 and 30 years old.
Step-by-Step Guide to Creating a Frequency Table on Excel
1. Organize Your Data
The first step is to organize your data into a range of cells. Each cell should represent a single observation or measurement. Ensure that the first row or column contains the class intervals, representing the ranges of values that the data falls into.
2. Create a Frequency Column
Next, create a column adjacent to your data range to count the frequency of each class interval. In this column, enter the following formula:
Cell |
Formula |
B2 |
=COUNTIF($A:$A, A2) |
This formula counts the number of cells in the data range (A:A) that are equal to the value in the corresponding class interval cell (A2). Drag this formula down the frequency column to count the frequency for each class interval.
3. Calculate the Cumulative Frequency
Finally, add a column to calculate the cumulative frequency for each class interval. This represents the total number of observations that fall within the class interval or any lower class intervals. In this column, enter the following formula:
Cell |
Formula |
C2 |
=SUM(B$2:B2) |
This formula sums the frequency of the corresponding class interval (B2) and all the frequencies above it (B$2:B2). Drag this formula down the cumulative frequency column to calculate the cumulative frequency for each class interval.
Counting the Frequency of Data Occurrences
Creating a frequency table in Excel allows you to quickly analyze the distribution of values in your dataset. By organizing the data into bins, or ranges of values, and counting the number of occurrences within each bin, you gain insights into the spread, central tendency, and potential patterns in your data.
Creating a Frequency Table
To create a frequency table in Excel, follow these steps:
1. Select the data range you want to analyze.
2. Go to the “Data” tab in the ribbon.
3. In the “Data Tools” group, click on “Data Analysis.”
4. Select “Histogram” from the list of analysis tools.
5. In the “Histogram” dialog box, set the “Input Range” to your selected data range.
6. Choose the “Bin Range” by specifying a start value, end value, and the number of bins. The number of bins determines the coarseness or fineness of your analysis.
7. Click “OK.”
Excel will generate a frequency table showing the bins, the frequency (count) of occurrences within each bin, and the cumulative frequency or percentage of occurrences.
Bins and Frequency
The distribution of values across bins provides valuable information about the data spread and potential patterns:
- Spread: The difference between the maximum and minimum values of the data. A wider spread indicates greater variability or dispersion.
- Skewness: The asymmetry of the distribution. A left-skewed distribution has more values towards the higher end of the range, while a right-skewed distribution has more values towards the lower end.
- Central Tendency: The “middle” of the distribution, which can be represented by the mean, median, or mode. A frequency table can indicate the tendency by showing the bin with the highest frequency of occurrences.
- Mode: The value that occurs most frequently. A frequency table can easily identify the mode as the bin with the highest count.
- Outliers: Unusual values significantly different from the rest of the data. Frequency tables can highlight outliers by showing bins with extremely low or high frequencies.
By interpreting the frequency table, you can gain valuable insights into the characteristics and patterns within your dataset, which can inform decision-making and further data analysis.
Using the FREQUENCY Function
The FREQUENCY function calculates the frequency of occurrence of each unique value in a range of cells. The syntax of the FREQUENCY function is as follows:
“`
=FREQUENCY(data_array, bins_array)
“`
Where:
For example, the following formula calculates the frequency of occurrence of each unique value in the range A1:A10.
“`
=FREQUENCY(A1:A10, A11:A20)
“`
The result of this formula would be an array of numbers, where each number represents the frequency of occurrence of the corresponding unique value in the range A1:A10.
Creating a Frequency Table
To create a frequency table, you can use the FREQUENCY function and the OFFSET function. The OFFSET function allows you to specify a cell offset from a given reference point. The following steps explain how to create a frequency table using the FREQUENCY and OFFSET functions:
-
Select the cell where you want to display the frequency table.
-
Enter the following formula into the cell:
=FREQUENCY(data_array, OFFSET(bins_array, 0, 0, ROWS(data_array), 1))
-
Press Enter.
-
The frequency table will be displayed in the selected cell.
The following table shows an example of a frequency table created using the FREQUENCY and OFFSET functions:
Value |
Frequency |
1 |
3 |
2 |
2 |
3 |
1 |
Creating a Bar Chart from the Frequency Table
Once you have created your frequency table, you can easily create a bar chart to visualize the data. Follow these steps:
1. Select the Data Range
Select the range of cells that contains your frequency table, including the category labels and the frequencies.
2. Insert a Bar Chart
Click on the “Insert” tab in the Excel ribbon and select “Bar Chart” from the “Charts” group. Choose the type of bar chart you want, such as a clustered bar chart or a stacked bar chart.
3. Customize the Chart
The chart will appear on your worksheet. You can customize it by changing the chart title, labels, and colors. To change the chart title, click on the chart and then click on the “Chart Title” field in the formula bar. To change the labels, click on the labels on the chart and type in the new labels.
4. Add Data Labels
To make the chart easier to read, you can add data labels to display the frequencies on top of each bar. Right-click on a bar and select “Add Data Labels” from the context menu.
5. Format the Chart
You can further enhance the appearance of your bar chart by formatting it. Here are some tips:
- Change the colors of the bars to make them more visually appealing.
- Add a legend to the chart to explain the meaning of the different colors.
- Add axes labels to clearly indicate what the x- and y-axes represent.
- Adjust the scale of the axes to ensure that the data is displayed accurately.
Calculating the Mode and Median
1. To calculate the mode, you need to find the value that appears most frequently in the dataset. In this example, the mode is 6, which appears three times.
2. To calculate the median, you need to find the middle value of the dataset when arranged in ascending order. In this example, the dataset can be arranged as {1, 2, 2, 3, 6, 6, 6}. Since there are an odd number of values, the middle value is the median, which is 6.
In a frequency table, the mode is the value with the highest frequency, while the median is the value that divides the dataset into two equal halves when arranged in ascending order. Both the mode and median are measures of central tendency, but the mode represents the most frequently occurring value, while the median represents the middle value.
Value |
Frequency |
1 |
1 |
2 |
2 |
3 |
1 |
6 |
3 |
Customizing the Frequency Table
Once you have created a basic frequency table, you can customize it to suit your needs.
Selecting the Data to Include
By default, Excel will include all of the data in the selected range in the frequency table. However, you can choose to include only specific data by using the “Filter” option in the “Data” tab. This allows you to filter out rows or columns based on specific criteria, such as removing empty cells or excluding certain values.
Changing the Bin Size
The bin size determines the width of each interval in the frequency table. By default, Excel will use a bin size of 1, but you can change this to any value you want. A smaller bin size will result in more intervals, while a larger bin size will result in fewer intervals.
Adding Custom Labels
You can add custom labels to the intervals in the frequency table by using the “Custom Labels” option in the “Frequency Table” dialog box. This allows you to specify specific labels for each interval, such as “Low”, “Medium”, and “High”.
Changing the Appearance
You can change the appearance of the frequency table by using the “Format” tab in the Excel ribbon. This allows you to change the font, color, and borders of the table. You can also add a title and chart to the table.
Sorting the Data
You can sort the data in the frequency table by frequency, value, or label. To sort the data, select the column you want to sort by and click the “Sort” button in the “Data” tab. You can choose to sort the data in ascending or descending order.
Adding a Histogram
A histogram is a graphical representation of the frequency table. You can add a histogram to the frequency table by clicking the “Histogram” button in the “Frequency Table” dialog box. The histogram will show the distribution of the data in the selected range.
Advanced Techniques for Frequency Analysis
8. Using Pivot Tables for Multi-Dimensional Analysis
Pivot tables offer a powerful tool for performing multi-dimensional frequency analysis. By arranging data in a pivot table, you can easily summarize and visualize frequencies across multiple variables. For example, you can create a pivot table to show the frequency of a variable (e.g., product sales) across different categories (e.g., region, product type). This allows you to identify trends and patterns that may not be immediately apparent from a simple frequency table.
To create a pivot table, select the data range and navigate to the “Insert” tab on the Excel ribbon. Click on the “PivotTable” button and specify the range for the pivot table. In the “PivotTable Fields” pane, drag and drop fields into the “Rows,” “Columns,” and “Values” sections to define the dimensions and measures of your analysis. You can also use filters to exclude specific data points and fine-tune your results.
Here’s an example of a pivot table that shows the frequency of product sales across different regions and product types:
Region |
Product Type |
Frequency |
East |
Electronics |
120 |
West |
Appliances |
80 |
North |
Furniture |
90 |
South |
Clothing |
110 |
This pivot table provides a quick overview of the sales distribution across different regions and product types. It allows you to easily identify top-selling products and regions, as well as areas with lower sales.
Troubleshooting Tips
Error: “Not enough memory”
If you receive this error, your spreadsheet may be too large for Excel to handle. Try closing other programs or reducing the size of your spreadsheet by removing unnecessary data or rows.
Another solution is to increase the amount of memory allocated to Excel. To do this, open Excel, click on “File” > “Options” > “Advanced”. Under the “Performance” section, select the “Advanced” button. In the “Virtual memory” section, increase the “Maximum memory usage” value to a higher number.
Error: “Cannot create pivot table”
This error can occur if your data does not meet the requirements for creating a pivot table. Make sure that your data is organized in a table format, with each column representing a different variable or category.
Error: “The formula you entered contains an error”
This error can occur if there is a syntax error in your formula. Check your formula carefully for any missing parentheses, commas, or other syntax errors.
Additional Tips
* When creating a frequency table, make sure to include all of the data that you want to analyze.
* If your data includes multiple categories, you can create a separate frequency table for each category.
* You can use the “Conditional Formatting” feature in Excel to highlight cells that meet certain criteria, such as cells that contain the most frequent values.
* You can use the “PivotTable” feature in Excel to create a more interactive and customizable frequency table.
Best Practices for Frequency Tables
To ensure accurate and informative frequency tables, follow these best practices:
1. Define Clear Categories
Establish precise categories for the data being analyzed. Ensure that each category is mutually exclusive and collectively exhaustive.
2. Use Standardized Values
Maintain consistency in the values used to represent data points. Avoid inconsistencies, such as using both “yes” and “Y” for the same category.
3. Include Absolute and Relative Frequencies
Display both the absolute frequency (count) and the relative frequency (percentage) for each category. This provides a comprehensive understanding of the distribution.
4. Sort Data Logically
Arrange the categories in a logical order, such as ascending or descending frequency, or by category type. This enhances readability and facilitates analysis.
5. Use Conditional Formatting
Apply conditional formatting to highlight specific values or ranges, making the table more visually appealing and easier to interpret.
6. Consider Grouping
If the data contains multiple variables, consider creating separate frequency tables for each variable or grouping categories into meaningful subgroups.
7. Use Pivot Tables
Excel’s pivot tables can be highly effective for creating and summarizing frequency tables, allowing for dynamic filtering and analysis.
8. Use Macros
To automate the creation and formatting of frequency tables, consider using Excel macros. This can save time and ensure consistency.
9. Include a Legend
If using symbols or colors to represent categories, include a clear legend to guide users’ understanding.
10. Extended Explanation of Relative Frequency Interpretation
Relative frequency helps assess the probability of occurrence within a category. It is calculated by dividing the absolute frequency of a category by the total number of observations in the dataset. Understanding relative frequency is crucial for insights:
Interpretation |
Relative Frequency Range |
Very frequent |
0.75 or higher |
Frequent |
0.50 – 0.74 |
Moderate |
0.25 – 0.49 |
Infrequent |
0.05 – 0.24 |
Very infrequent |
0.04 or lower |
This understanding enables informed decisions and predictions based on the frequency of occurrences in the analyzed data.
How to Create a Frequency Table in Excel
Excel is a powerful tool that can be used for a variety of data analysis tasks, including creating frequency tables. A frequency table is a table that shows the number of times each value in a data set occurs. This can be useful for identifying patterns and trends in the data.
Here are the steps on how to create a frequency table in Excel:
- Enter your data into a range of cells.
- Select the range of cells that contains your data.
- Click on the “Data” tab in the ribbon.
- Click on the “Data Analysis” button in the “Analyze” group.
- Select “Frequency” from the list of data analysis tools.
- Click on the “OK” button.
Excel will then create a frequency table that shows the number of times each value in your data set occurs.
People Also Ask about How to Create a Frequency Table in Excel
How can I create a frequency table for multiple columns?
To create a frequency table for multiple columns, you can use the CONCATENATE function to combine the values in the different columns into a single column. You can then create a frequency table for the concatenated column.
How can I create a frequency table that shows percentages?
To create a frequency table that shows percentages, you can use the FREQUENCY function to calculate the frequency of each value in your data set. You can then use the PERCENTAGE function to calculate the percentage of each value in the data set.
How can I create a frequency table that shows cumulative frequencies?
To create a frequency table that shows cumulative frequencies, you can use the SUBTOTAL function to calculate the cumulative frequency of each value in your data set. You can then use the OFFSET function to create a range of cells that contains the cumulative frequencies.